Uber Freight: Artificial Intelligence Serving Logistics and Supply Chain Management
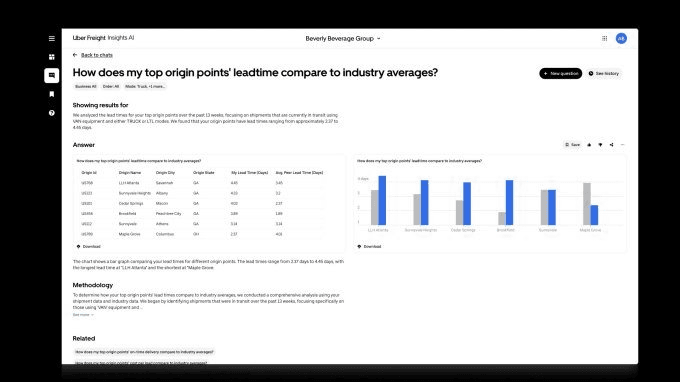
Listen to this article
Uber Freight: Artificial Intelligence Serving Logistics and Supply Chain Management
As globalization, demand volatility, and cost pressures make logistics management increasingly complex, the digital transformation of supply chains is no longer just a competitive advantage: it has become a strategic necessity. In this context, Uber Freight is becoming a key player in the market by resolutely betting on artificial intelligence (AI) to digitize, optimize, and provide visibility across the entire logistics cycle. How does AI applied to logistics reinvent planning, flow management, and decision-making models? What synergies does it offer with no-code platforms, automation, and data-driven architectures? And what lessons can be drawn for mid-sized companies and large corporations seeking operational modernization?
This article provides an in-depth analysis of Uber Freight’s strategy, the impacts of AI on logistics, and key integration points for an agile, responsive, and data-driven supply chain.
AI as a Driver of Digitization and Logistics Optimization
The digitization of supply chain processes is accelerating at an unprecedented pace thanks to AI. Initially positioned as a logistics broker, Uber Freight has developed a range of artificial intelligence solutions within a few years to excel in three key areas: operational efficiency, predictive planning, and real-time visibility.
Reducing Logistics Costs and Enhancing Operational Efficiency
One of the main promises of AI in the supply chain is cost rationalization. Uber Freight uses machine learning models capable of continuously comparing routes, carriers, contracts, and processed volumes. Algorithms evaluate freight options based on prices, deadlines, and availability, automatically generating suggestions to choose the best cost-quality-time combination. For client companies, this results in measurable reductions in transport costs and productivity gains, notably by reducing the time spent manually analyzing disparate data.
Predictive Inventory Management and Flow Optimization
Logistics planning becomes more proactive with tools such as Uber Freight’s Insights AI, which consolidates and analyzes data from multiple systems (ERP, TMS, carrier platforms) to anticipate shortages, bottlenecks, or overstocked areas. The use of a specialized Large Language Model (LLM) enables the formulation of complex questions, breaking down information silos and immediately identifying underperforming routes, occasional excess costs, or underutilized logistics partners. This frees operational teams, who can now focus on proactive rather than reactive resolution of supply chain incidents.
End-to-End Visibility and Traceability
The real-time integration of data flows using AI provides cross-functional visibility of all logistics operations: shipment location, compliance with deadlines, alerts on deviations from contractual KPIs, and so on. The decision-making chain accelerates: whereas analyzing a logistics problem previously required several weeks and back-and-forth between departments, AI brings incidents and their resolution much closer together. This responsiveness is crucial in markets characterized by tight margins and high competitive pressure.
Synergies with No-Code Digitization and Data-Driven Platforms
Adaptive Automation via No Code/Low Code Tools
Integrating AI solutions into no-code or low-code environments makes digital transformation accessible to a wider range of business users. Modular logistics platforms allow AIs to be plugged in, then orchestrate tasks such as automated shipment tracking, report generation, or alert management with minimal IT team intervention. This lowers the technical barrier to innovation and makes agile supply chain management easier.
Interoperability and Modular Architectures
The open architectures promoted by Uber Freight (APIs, connectors, cloud platforms) correspond with the data-centric approach favored by most mid-sized and large companies, which are seeking to unify their information flows. AI then integrates as a value-added “building block” within an ecosystem already comprising ERPs, WMS (warehouse management systems), TMS (transport management systems), and analytical platforms. This interoperability accelerates the move towards data-driven supply chain models—essential for long-term competitiveness.
Data Governance and Security
The widespread adoption of AI highlights the issue of data quality and governance. To deliver truly actionable insights, AI models need access to coherent, structured, and reliable datasets. Companies must invest in building their data foundations and training teams in AI ethics to ensure compliance and minimize the risks of bias, hallucination, or erroneous interpretations.
Concrete Use Cases: From Intelligent Supervision to Proactive Management
Carrier Performance Management
Take the example of Colgate-Palmolive, which uses Uber Freight to monitor the engagement and performance of its thousands of carriers. Previously, identifying partners failing to meet their contractual quotas or timelines was a daunting challenge due to fragmented data. Now, AI automatically analyzes volumes, signals anomalies (non-compliance, excess costs, delays), and proposes personalized corrective actions (portfolio rebalancing, renegotiation, replacement recommendations).
Multi-Criteria Optimization and Financial Alerts
Insights AI also enables real-time detection of inefficiency pockets, such as routes that are more expensive than average, abnormal expenditures on specific lanes, or opportunities to pool flows. Aggregating these micro-suggestions can result in annual savings of millions of euros internationally, while improving customer satisfaction through controlled lead times. Feedback from the field highlights that finance departments are enthusiastic about these tools, which make budget management much more dynamic and automated.
Continuous Improvement and Risk Anticipation
Beyond reducing costs or ensuring contractual compliance, AI drives continuous improvement in supply chain management. Companies can anticipate disruptions (weather events, undercapacity, strikes) using predictive models that cross-reference industry, historical, and contextual weak signal data. This allows scenario planning and real-time adaptation—a key advantage in times of volatility.
Integration into the Digital Transformation Strategy and Agile Management
Uber Freight’s experience highlights a key point for any digital transformation initiative: AI should not be seen as an isolated “black box,” but as a modernization catalyst within an overall, modular, and data-driven approach.
Facilitating Change and Skills Development
Client feedback emphasizes the importance of training (for example, the ethical approach required at Colgate-Palmolive) and developing teams’ familiarity with AI—essential conditions for field adoption. No-code plays a role here as an adoption driver, allowing operational staff to create tailor-made solutions without relying exclusively on technical experts.
Opening Up to New Management Models
The widespread use of AI in logistics, combined with automation and dematerialization, paves the way for agile management models (hybrid decision systems, proactive alerts, near real-time reporting) and faster experimentation thanks to adaptable architectures.
Limits, Challenges, and Perspectives
While the potential of AI in logistics is considerable, its large-scale deployment raises several challenges:
- Data quality: Consistency and completeness remain critical to avoid bias and ensure the reliability of automated suggestions.
- Complexity of legacy systems: The heterogeneity of legacy systems sometimes hinders the seamless integration of AI solutions.
- Operational acceptability: Moving to a data-driven model involves a cultural shift that requires support and training.
- Regulatory and ethical challenges: AI governance and data confidentiality management are sensitive topics.
Nevertheless, Uber Freight is paving the way for a profound transformation, where artificial intelligence, no-code automation, and data strategy converge to create a resilient, responsive, and intelligently managed supply chain.
Conclusion
Uber Freight is not merely emblematic of the AI shift in logistics: its approach reflect a structural evolution toward connected, transparent, and intelligent supply chains. For mid-sized and large companies, integrating artificial intelligence tools into an overarching digital strategy that combines modularity, interoperability, and data-driven management is a guarantee of lasting competitiveness. The challenge no longer lies in the technology, which has now matured, but in the ability to rethink structures, processes, and skills to make the most of this new logistics paradigm.
Keywords: Uber Freight, artificial intelligence, logistics, supply chain, digital transformation, process optimization, NoCode, automation, data-driven, predictive management
Tags
Articles connexes
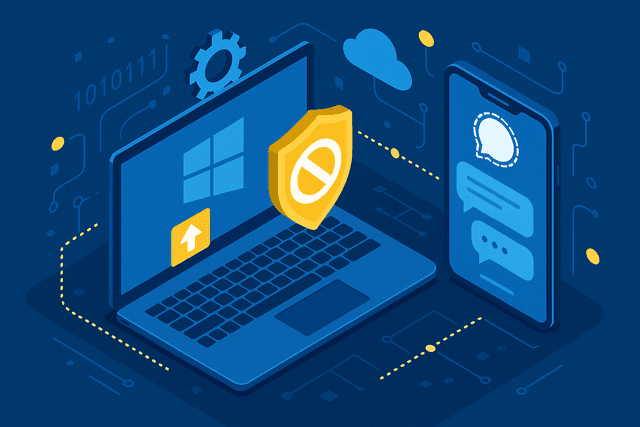
Signal’s Screenshot Blocking Update: Bolstering Enterprise Security and Digital Transformation
Explore Signal screenshot blocking and its impact on enterprise security messaging and digital transformation security with the latest Signal Windows 11 update.
Read article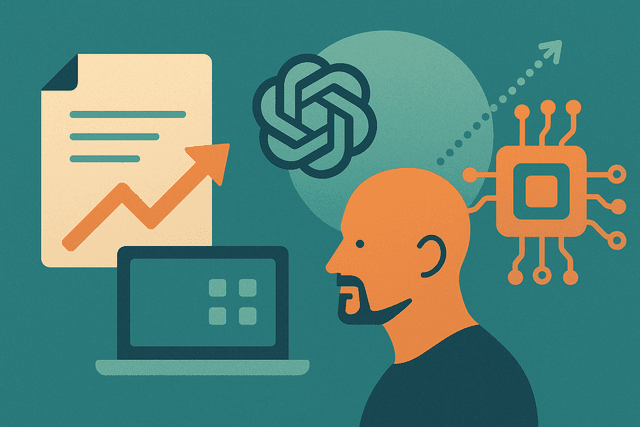
OpenAI’s $6.5B Bet on Jony Ive’s Startup: Charting New Frontiers for AI in Hardware
Explore OpenAI’s $6.5B acquisition of Jony Ive’s AI startup, driving hardware advances and integrating AI into next-gen devices for a smarter future.
Read article